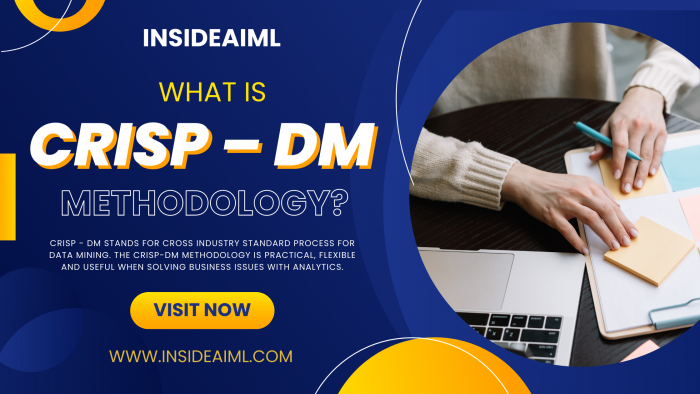
Crisp dm is a consistent process for data mining that can be applied across domains. The crisp dm method is feasible, flexible, and useful for applying analytics to business challenges.
Crisp dm is a data mining method. Industry giants like Daimler-Benz, ISL, NCR, and OHRA were among its original members, and it has been in use since 1996. These companies have deployed about 200 data mining users and tools for this strategy. This method is accessible to everyone with an internet connection because of the lack of intellectual property protection.
Do you see any advantages?
The crisp dm framework provides a roadmap, best practices, and frameworks to help businesses improve the efficiency and effectiveness of their data mining initiatives.
Knowing How to Do Business
The first phase, "Business Knowledge," involves breaking down the project into manageable chunks using data mining technologies after starting with a business goal or understanding.
There are four main objectives of business education, and they are as follows:
Finding out what the organization wants to accomplish is the first step. This is when we get down to brass tacks and learn about the inner workings of your business and the impetus behind your project.
Compile a list of assumptions and run a cost-benefit analysis to determine how serious of a problem you're dealing with.
We plan out data mining strategies for the entire organization or department.
Include a detailed breakdown of the project's timeline, resources, and deliverables.
Statistical Knowledge
Data collecting is the initial step in the second phase, data understanding, which comprises familiarizing oneself with the data and making inferences based on the data's quality and the knowledge currently at hand. If we have access to any intriguing data sets, we can use the information buried within them to formulate a working hypothesis.
There are four main aims of data understanding, and they are as follows.
Before anything else, there is the "data collecting" phase, so make sure to keep track of any problems you encounter.
Here, we examine the underlying data to see if there were any problems during collection, and we can also see what data formats are available, how much data we have, and what kind of data it is, as well as identify fields and records on tablets.
Third, conduct exploratory data analysis, which comprises writing a data exploration report outlining your preliminary findings and hypotheses.
The fourth step is to check the accuracy of the information you have gathered by looking for things like missing attributes, blank fields, and misspelled values. Data inconsistencies can also be identified and documented.
Preparing Information for Analysis
The third phase, "data preparation," might create the final dataset for modeling if the data is good. This phase gathers all data and chooses a final set of facts for modeling.
Easy tasks include:
So that we may get things rolling: Choose - Pick Which Data Will Be Used
Next, we verify that the data is complete and accurate by looking for things like missing attributes and typos in the spelling.
The third phase is called "Build," and it entails making brand-new records or outlining specific qualities.
In the final phase, "integrate," information from several databases is compiled and merged.
Modeling
To model something, we first propose a variety of modeling approaches, then pick one at random and run with it to test its viability and investigate the alternatives.
The 3 primary roles of a model are as follows:
Choose a Version
Experiment with the model.
Modeling Do a model analysis.
Evaluation
To begin, we formulate and pursue our company's objectives. We then conduct process reviews and create assessment forms. These become corporate standards.
Deployment
Deployment, the last phase, involves handing off the report and moving forward with the project or starting company operations.
The most vital steps are as follows:
Get Ready to Deploy
Follow up with people;
Compile a final report and submit it.
Phase Four of the Project Evaluation
Hence, we got an education about crisp dm and its methods. The next articles will cover crisp dm.
There are four main objectives of business education, and they are as follows:
Finding out what the organization wants to accomplish is the first step. This is when we get down to brass tacks and learn about the inner workings of your business and the impetus behind your project.
Compile a list of assumptions and run a cost-benefit analysis to determine how serious of a problem you're dealing with.
We plan out data mining strategies for the entire organization or department.
Include a detailed breakdown of the project's timeline, resources, and deliverables.
Knowing How to Do Business
The first phase, "Business Knowledge," involves breaking down the project into manageable chunks using data mining technologies after starting with a business goal or understanding.
There are four main objectives of business education, and they are as follows:
Finding out what the organization wants to accomplish is the first step. This is when we get down to brass tacks and learn about the inner workings of your business and the impetus behind your project.
Compile a list of assumptions and run a cost-benefit analysis to determine how serious of a problem you're dealing with.
We plan out data mining strategies for the entire organization or department.
Include a detailed breakdown of the project's timeline, resources, and deliverables.
Statistical Knowledge
Data collecting is the initial step in the second phase, data understanding, which comprises familiarizing oneself with the data and making inferences based on the data's quality and the knowledge currently at hand. If we have access to any intriguing data sets, we can use the information buried within them to formulate a working hypothesis.
There are four main aims of data understanding, and they are as follows.
Before anything else, there is the "data collecting" phase, so make sure to keep track of any problems you encounter.
Here, we examine the underlying data to see if there were any problems during collection, and we can also see what data formats are available, how much data we have, and what kind of data it is, as well as identify fields and records on tablets.
Third, conduct exploratory data analysis, which comprises writing a data exploration report outlining your preliminary findings and hypotheses.
The fourth step is to check the accuracy of the information you have gathered by looking for things like missing attributes, blank fields, and misspelled values. Data inconsistencies can also be identified and documented.
Preparing Information for Analysis
The third phase, "data preparation," might create the final dataset for modeling if the data is good. This phase gathers all data and chooses a final set of facts for modeling.
Easy tasks include:
So that we may get things rolling: Choose - Pick Which Data Will Be Used
Next, we verify that the data is complete and accurate by looking for things like missing attributes and typos in the spelling.
The third phase is called "Build," and it entails making brand-new records or outlining specific qualities.
In the final phase, "integrate," information from several databases is compiled and merged.