As businesses evolve, data has become a critical asset for guiding strategic decision-making and driving innovation. However, the volume of data generated every day can overwhelm even the most sophisticated enterprises. This problem is addressed through the concept of Decision Intelligence (DI), which provides a methodology for transforming raw data into actionable insights. Decision Intelligence combines data science, artificial intelligence (AI), human reasoning, and surrounding circumstances to improve decision-making. It provides an effective, comprehensive strategy in bridging the gap between data analytics and business strategy, ensuring that leaders have the necessary information to make reliable decisions, minimize risks, and maximize emerging opportunities.
The Shift from Data to Decision Intelligence
Data-driven decision-making isn't a new concept. Businesses have utilized analytics for years for competitive advantage, operational efficiency, and enhanced customer experiences. Traditional data analytics deals mostly with what has happened and why it happened. It leaves gaps in predicting future outcomes and the prescription of action toward an optimal resolution. Decision Intelligence aims to fill those gaps through the integration of predictive and prescriptive analytics, machine learning, and other cognitive technologies. It goes beyond the analysis of information and focuses on the context in which decisions are made. This structure acknowledges that data alone is insufficient; it requires human intuition, informed judgment, and organizational objectives to bring meaningful change.
For example, a retail company that traditionally uses analytics to determine a decline in sales for a particular product category. Without decision intelligence, this company may not uncover the reason for the decline, as it could be because of various factors like changing customer preferences, supply chain issues, or increased competition. However, with DI, the same company can integrate data from multiple sources and apply machine learning with real-time market analysis to understand the full picture. With these advanced technologies, businesses can refine their marketing, alter pricing strategies, or optimize inventory and supply levels to enable higher returns.
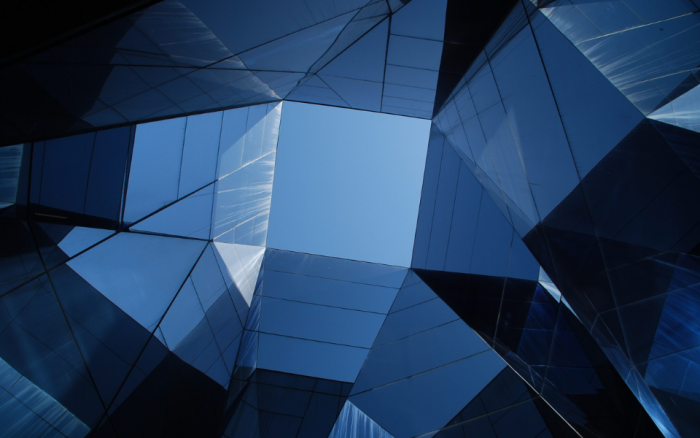
Core Components of Decision Intelligence
Data, models, and context are the three pillars of Decision Intelligence. Each of these components helps in the conversion of data into actionable insights:
Data: This is the base of every Decision Intelligence System (DIS). It comprises both structured data, such as sales and financial figures, as well as unstructured data, such as customer reviews and social media posts that offer insights into business activities. Modern businesses utilize data from various sources: internal databases, customer interactions, IoT devices, and third-party platforms. This data must be accurate, reliable, and available in real time, as it directly impacts decision-making
Models: Advanced analytical models can identify patterns, recommend actions, and predict outcomes. From basic statistical analyses to complex machine learning algorithms with AI, these models can be as simple or advanced as they need to be. The chosen models depend on the business problem at hand, the type of data available, and the intended results. For example, predictive models would estimate customer demand, while prescriptive ones would recommend pricing strategies depending on the constantly changing parameters of the market.
Context: Context is the distinguishing feature of decision intelligence compared to more traditional forms of analytics. Context guarantees that the information produced is useful, precise, and synced with the relevant frameworks. Even with the most advanced models, results become faulty, and decision-making is hindered without sophisticated context, which leads to poor decision-making.
Real-World Applications of Decision Intelligence
Most leading companies have adopted DI to automate business processes, improve customer interactions, and maximize profits. Here are a few notable examples:
Supply Chain Optimization: Companies like Amazon and Walmart incorporate DI to forecast demand, manage inventory, and streamline logistics, all of which cut operational costs while enhancing the customer’s experience.
Financial Services: Banks and financial services companies use DI to evaluate credit risk, identify fraudulent transactions, and customize offerings to customers. This improves profits and customer trust.
Healthcare: Hospitals and other healthcare providers use DI to enhance patient outcomes, allocate resources efficiently, and reduce operational inefficiencies. For instance, predictive analytics can help identify patients who are at risk, enable targeted interventions, and lower hospital readmission rates.
Marketing and Customer Insights: Companies like Netflix and Spotify use DI to tailor experiences for users, predict churn, and optimize marketing campaigns, which enhances customer retention and increases lifetime value.
Challenges in Implementing Decision Intelligence
Though Decision Intelligence has its benefits, it's also important to acknowledge the challenges in its implementation. These include:
Data Quality and Integration: Most organizations face difficulties in merging data from different sources, leading to gaps, misinformation, or inaccurate insights. Data Intelligence (DI) works best with clean and high-quality data. This is where DataOps Services is critical in automating the governance of data across various platforms..
Skills Gap: Creating and managing DI systems requires specialized skills in data science, machine learning, and business strategy. Many companies struggle to fill these crucial roles.
Cultural Resistance: Moving from an intuition-based decision-making framework to a data-centric approach can bring significant cultural challenges that require strong leadership and effective change management.
Ethical and Privacy Concerns: As DI systems advance, organizations have to deal with complicated ethical and privacy considerations related to data practices. They need to ensure compliance with laws such as GDPR and CCPA.
The Future of Decision Intelligence: From Insight to Autonomous Decision-Making
As Decision Intelligence evolves, the next frontier lies in enabling autonomous decision-making powered by AI agents. These systems will not only analyze and interpret data but also make decisions with minimal human intervention, especially for repetitive, time-sensitive tasks. Enterprises are exploring agentic AI and generative AI to build adaptive DI systems that learn continuously from outcomes and context. This advancement will drive faster responses to market dynamics, greater operational agility, and smarter resource utilization. Businesses that invest early in intelligent automation and decision intelligence platforms will lead the way in achieving scalable, real-time, and impact-driven transformation.
Conclusion
In modern businesses, data is an asset, but the only way its benefit is fully reaped is through Decision Intelligence. Combining data with advanced analytics and technology allows an organization to properly manage decisions, which facilitates scaling the business, mitigating risks, and leading to innovation. As organizations start to adopt Decision Intelligence, they begin to move away from traditional methods of analytics to more innovative and broad-scope predictive and prescriptive strategies. This shift in mindset is not just about data; it’s about transforming data into actionable insights that can take a business to the next level. The evolution of DI will greatly assist in overcoming challenges, revealing new opportunities, and maintaining a competitive edge in the industry.