In the rapidly evolving world of data science, businesses are constantly seeking ways to gain a competitive edge. One of the most powerful tools in a data scientist's toolkit is A/B testing and experimentation. These methodologies allow organizations to make data-driven decisions, optimize processes, and ultimately improve their bottom line. In this article, we will delve into the world of A/B testing and experimentation, exploring its key principles, applications, and the critical role it plays in data-driven decision-making. We will also touch upon the importance of proper Data Science training and other locations to harness the full potential of these techniques.
Understanding A/B Testing and Experimentation
A/B testing, also known as split testing, is a method of comparing two versions of a web page, app, or any digital product to determine which one performs better. The process involves splitting the audience into two groups: Group A is shown the original version (the control group), and Group B is shown a slightly modified version (the experimental group). The goal is to identify which version produces superior results, whether it be higher click-through rates, increased conversion rates, or other key performance indicators.
Experimentation, in the context of data science, extends beyond A/B testing. It encompasses a broader range of activities aimed at exploring, testing, and validating hypotheses to gain insights and drive decision-making. A/B testing is just one specific type of experimentation used in this process.
The Steps of A/B Testing and Experimentation
A/B testing, also known as split testing, is a systematic approach to experimentation that involves several key steps. The process begins with the identification of a clear objective. This initial step is crucial as it establishes what you aim to achieve through the test. It could be an increase in sales, higher user engagement, or an improved user experience.
Following the definition of the objective, the next step is hypothesis generation. You formulate a hypothesis that outlines the expected impact of the changes you intend to make. This hypothesis serves as a guiding framework for the entire testing process, setting the direction for your experiment.
Randomization is another essential component of A/B testing. To ensure the validity of your results, you randomly assign users or subjects to two groups: the control group (A) and the experimental group (B). This randomization helps ensure that the groups are comparable, minimizing bias in the results.
Once the groups are established, you proceed with implementation. Changes or modifications are applied to the experimental group (B), while the control group (A) remains unchanged. This setup allows you to isolate the effects of the specific changes you are testing. Data collection is a critical phase where you gather data on the performance of both groups. You track relevant metrics such as conversion rates, bounce rates, or revenue. The collected data forms the basis for your analysis.
Statistical analysis comes into play next. Using various statistical techniques, you compare the performance of the control group (A) with that of the experimental group (B). This analysis helps determine whether the observed differences are statistically significant or merely the result of chance variation.
Based on the results of the statistical analysis, you draw conclusions about whether the changes had a significant impact on the desired outcome. If the experimental group outperforms the control group, it may be time to implement the changes on a larger scale. However, if the results are inconclusive or if the control group performs better, you may need to revisit your approach and refine your hypotheses. In summary, A/B testing is a structured process that begins with defining objectives and formulating hypotheses, followed by randomization, implementation, data collection, statistical analysis, drawing conclusions, and, if successful, implementing findings on a broader scale. It's a powerful method for optimizing various aspects of business and user experiences through empirical experimentation.
Applications of A/B Testing and Experimentation
A/B testing and experimentation have a wide range of applications across various industries. Here are a few examples:
1. E-Commerce: Online retailers use A/B testing to optimize product pages, checkout processes, and marketing strategies. By experimenting with different layouts, product descriptions, or pricing strategies, they can increase sales and revenue.
2. Content Marketing: Content creators and marketers use A/B testing to determine which headlines, images, or call-to-action buttons are most effective in driving user engagement and conversions.
3. Mobile Apps: App developers frequently employ A/B testing to improve user retention, app usability, and in-app purchases. By experimenting with different features or user interfaces, they can enhance the user experience.
4. Healthcare: In healthcare, experimentation is used to test the effectiveness of new treatments, drug dosages, and patient care protocols. This helps healthcare providers make evidence-based decisions that improve patient outcomes.
5. Finance: Financial institutions utilize A/B testing to optimize their online banking platforms, trading interfaces, and customer service channels. These tests can lead to increased user satisfaction and loyalty.
The Role of Data Science Training
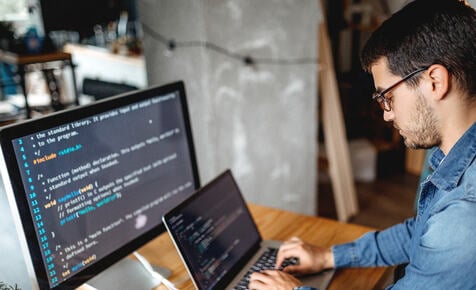
While A/B testing and experimentation are powerful tools, their effective implementation requires a deep understanding of data science concepts, statistical analysis, and experimental design. This is where proper Data Science training in Lucknow, Meerut, Noida, Delhi and other locations becomes crucial.
Data Science training programs provide aspiring data scientists with the knowledge and skills needed to excel in this field. Here are some ways in which Data Science training can enhance the effectiveness of A/B testing and experimentation:
1. Statistical Expertise: Data science training equips individuals with a solid foundation in statistics, which is essential for designing experiments, conducting hypothesis tests, and interpreting results accurately.
2. Data Handling: Data scientists are trained in data collection, cleaning, and preprocessing, ensuring that the data used in experiments is reliable and free from biases.
3. Machine Learning: Machine learning techniques can be integrated into experimentation to create more sophisticated models for predicting outcomes and personalizing user experiences.
4. Data Visualization: Data scientists are skilled in data visualization, allowing them to present results in a clear and compelling manner, making it easier for decision-makers to understand the findings.
5. Experimental Design: Data science training covers experimental design principles, helping individuals create experiments that are robust and capable of yielding meaningful results.
6. Ethical Considerations: Training programs also emphasize ethical considerations in data collection and experimentation, ensuring that experiments are conducted in a responsible and unbiased manner.
There is a growing demand for professionals with expertise in data science. Organizations across industries are recognizing the value of data-driven decision-making, and they are actively seeking individuals who can leverage A/B testing and experimentation to drive business growth.
Challenges and Considerations in A/B Testing and Experimentation
While A/B testing and experimentation offer significant benefits, they are not without challenges and considerations. Here are a few key points to keep in mind:
1. Sample Size: Ensuring that the sample size for each group is sufficient is essential for obtaining statistically significant results. Inadequate sample sizes can lead to unreliable conclusions.
2. Duration of Testing: The duration of an A/B test should be carefully chosen to account for seasonality and other external factors that may impact results.
3. Multiple Testing: Conducting multiple tests simultaneously can lead to false positives, so it's important to adjust for multiple comparisons when analyzing results.
4. Interpreting Results: Statistical significance does not always equate to practical significance. It's crucial to consider the real-world impact of observed differences.
5. Bias and Ethics: Be mindful of potential biases in data collection and experimentation, and ensure that experiments are conducted ethically and in compliance with privacy regulations.
Conclusion
A/B testing and experimentation are invaluable tools in the realm of data science. They empower organizations to make informed decisions, optimize processes, and improve user experiences. However, to harness their full potential, individuals must undergo proper Data Science training to acquire the necessary skills in statistics, data handling, and experimental design.
As the demand for data-driven insights continues to grow, the role of data scientists proficient in A/B testing and experimentation will become increasingly critical. These professionals will play a pivotal role in shaping the strategies and outcomes of businesses and organizations across industries, ultimately driving success in the ever-evolving world of data science.